top of page
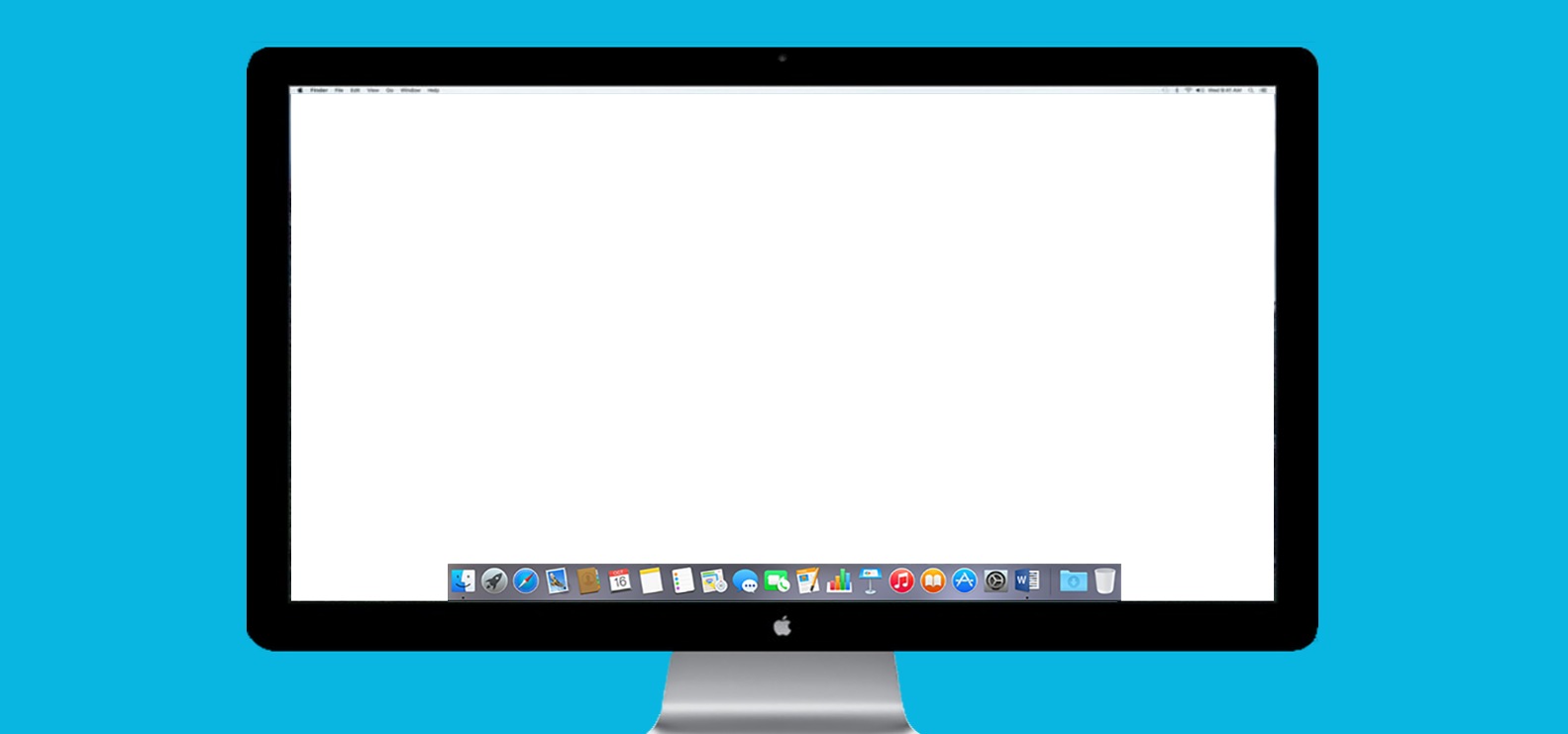
Lecture 36: Statistics
Lecture 37: Conclusion
Bayes' Rule
(070616)
Baye's Rule
A rule for updating probabilities based on new evidence Provided with P(A), P(B), and P(B|A)... compute P(A|B) P(A|B) = P(B|A) * P(A) / P(B)
P(A) : Prior probability of some event before evidence
P(B|A) : Likelihood of evidence given this event
P(B) : Probability of evidence in general
P(A|B) : Posterior probability of event after evidence
Main Uses of Confidence Intervals
(070616)
Confidence Intervals
● To estimate a parameter:
○ You can just take the middle 95% of the estimates if you don’t want to deal with subtracting deviations
● Regression prediction: predict y based on a new x
● To test the null hypothesis that the parameter is equal to a specified value:
○ In the regression model, test whether the slope of the true line is 0
Tests of Hypotheses
(070616)
Hypothesis
● Null:
The observation is “just due to chance”. Need to say exactly what is due to chance, and
what the hypothesis specifies.
● Alternative:
The null isn’t true; something other than chance is going on.
P-value
(070616)
P-value
● The chance, under the null hypothesis, that the test statistic comes out like the one in the sample or more extreme.
● If this chance is small, then:
○ If the null is true, something very unlikely has happened.
○ Conclude that the data support the alternative hypothesis better than they support the null.
Comparing
Two Numerical Samples
(070616)
Numerical Samples
● Null:
The two samples come from the same underlying distribution in the population.
● Method:
○ Bootstrap A/B test
○ Test statistic: difference between means
Comparing
Two Categorical Samples
(070616)
Categorical Samples
● Null:
The two samples come from the same underlying distribution in the population
(e.g. “distribution of employment status is the same for married and unmarried men”).
● Method:
○ Permutation test
○ Test statistic: total variation distance between the distributions of the two samples
One Categorical Sample
(070616)
1-Categorical Samples
● Null:
The sample was drawn at random from a specified distribution
(e.g. known from Census data, “the coin is fair”, etc).
● Method:
○ Simulation: Generate samples from the distribution specified in the null.
○ Test statistic: total variation distance between distribution in sample and distribution
specified in the null.
bottom of page